Main Effect Factorial Design
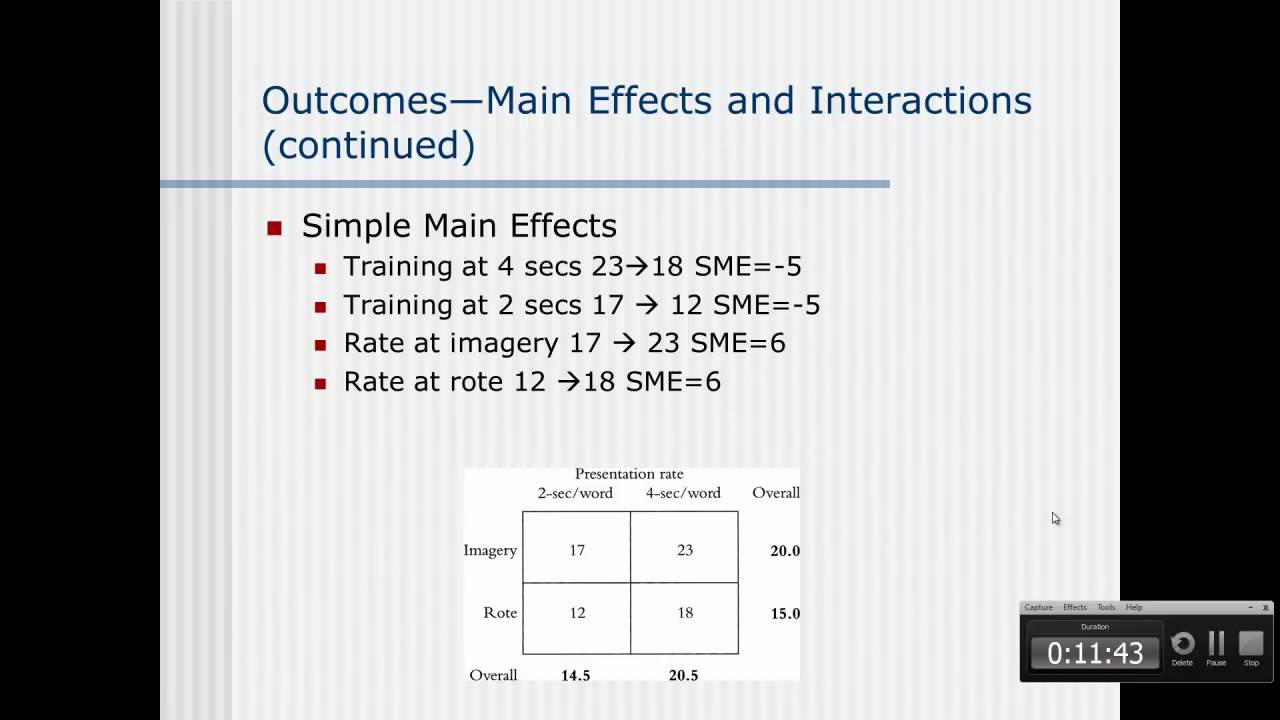
Understanding the intricacies of experimental design is crucial in various fields, including psychology, biology, and social sciences. Among the array of designs used to investigate the effects of variables, the main effect factorial design stands out for its ability to examine the impact of independent variables on a dependent variable. This design is particularly useful when researchers aim to understand not just the individual effects of variables but also how these variables interact with each other.
At its core, a main effect factorial design involves the manipulation of two or more independent variables to observe their effects on a dependent variable. Each independent variable is referred to as a factor, and these factors are varied across different levels. For instance, if a researcher is interested in how both the lighting conditions (bright vs. dim) and the noise levels (loud vs. quiet) in a workspace affect productivity, they would use a 2x2 factorial design. Here, “2x2” denotes that there are two factors (lighting conditions and noise levels), each with two levels.
Key Components of Main Effect Factorial Design
Factors and Levels: The design is characterized by the number of factors and the levels of each factor. A 2x2 design, for example, includes two factors, each manipulated at two levels. This setup allows researchers to assess the main effect of each factor (how each factor affects the outcome variable on its own) and the interaction effect (how the factors combine to affect the outcome variable).
Treatment Conditions: Each unique combination of factor levels constitutes a treatment condition. In a 2x2 design, there would be four treatment conditions: (1) bright lighting and loud noise, (2) bright lighting and quiet noise, (3) dim lighting and loud noise, and (4) dim lighting and quiet noise.
Randomization: Participants are randomly assigned to treatment conditions to minimize bias and ensure that the groups are comparable in terms of variables not being manipulated.
Control: While factorial designs can include control conditions (where none of the factors are manipulated), the main effect design focuses on the manipulation of specified factors to understand their effects.
Analyzing Main Effects and Interactions
The main effect of a factor refers to the change in the dependent variable that occurs when that factor is manipulated, averaged across all levels of the other factor. For example, if we find a main effect of lighting conditions on productivity, it means that, on average, productivity differs significantly between bright and dim lighting conditions, regardless of the noise level.
Interactions occur when the effect of one factor depends on the level of another factor. In our example, an interaction between lighting and noise would mean that the impact of lighting conditions on productivity varies depending on the noise level. For instance, perhaps bright lighting improves productivity more in quiet than in loud conditions.
Advantages of Main Effect Factorial Design
- Efficiency: Allows for the examination of multiple factors and their interactions in a single experiment, making it more efficient than conducting separate experiments for each factor.
- Comprehensive Understanding: Provides a nuanced view of how variables interact, which is crucial for understanding complex phenomena.
- External Validity: The randomized assignment and control over variables enhance the internal validity, but the artificial setting might affect external validity.
Challenges and Considerations
- Complexity: As the number of factors and levels increases, the design becomes more complex, requiring more participants and resources.
- Interpretation: Analyzing and interpreting interactions can be challenging, especially in higher-order factorial designs.
- Assumptions: Statistical analyses of factorial designs assume normality, homogeneity of variance, and independence of observations, which must be checked and addressed if violated.
In conclusion, the main effect factorial design is a powerful tool for researchers seeking to understand the effects of multiple variables on an outcome. By carefully designing and analyzing experiments with this approach, researchers can gain a deeper insight into the complex interactions that underlie many phenomena, ultimately contributing to more informed decision-making and theory development.