Deirdre De La Cruz
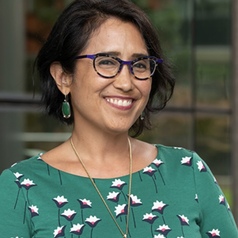
Deirdre De La Cruz, a name that evokes a sense of mystique and intrigue, much like the complex, ever-evolving world of artificial intelligence, which has been transforming the way we live, work, and interact with one another. As we delve into the realm of AI, it becomes increasingly clear that understanding its potential, limitations, and implications is crucial for navigating this new landscape. This exploration will take us through the historical evolution of AI, its current applications, and the future trends that are expected to shape not just technology, but society as a whole.
Historical Evolution: From Origins to Present
The concept of artificial intelligence has its roots in ancient myths and legends, where beings created by gods or humans possessed intelligence and capabilities rivaling those of their creators. However, the modern study of AI began in the mid-20th century, with pioneers like Alan Turing, Marvin Minsky, and John McCarthy laying the foundational groundwork. Turing’s 1950 paper, “Computing Machinery and Intelligence,” proposed what is now known as the Turing Test—a measure of a machine’s ability to exhibit intelligent behavior equivalent to, or indistinguishable from, that of a human.
Over the decades, AI has seen periods of significant advancement followed by periods dubbed “AI winters,” where funding and interest waned due to the failure of achieving expected results. The field has diversified into several subfields, including machine learning (ML), natural language processing (NLP), computer vision, and robotics, each contributing uniquely to the broader tapestry of AI research and application.
Current Applications: Transforming Industries
Today, AI is not just a concept of science fiction but a reality that permeates our daily lives. From the personal assistants like Siri, Alexa, and Google Assistant that we interact with in our homes, to the algorithms that suggest products on e-commerce platforms, AI is ubiquitous. In healthcare, AI is used for diagnosis, drug discovery, and personalized medicine. In finance, it powers trading platforms, detects fraud, and provides investment advice. The automotive industry is on the cusp of a revolution with autonomous vehicles, while education sees the rise of adaptive learning systems.
These applications are not merely enhancements; they represent fundamental shifts in how industries operate, creating new business models, and challenging traditional practices. AI is not just about automation; it’s about augmentation—enhancing human capabilities, improving accuracy, and enabling speeds that are beyond human capacity.
Future Trends: Challenges and Opportunities
Looking ahead, several trends are poised to reshape the AI landscape. The integration of AI with the Internet of Things (IoT) will create smart environments that can adapt and respond to human needs in real-time. Edge AI, which processes data closer to where it is generated, will reduce latency and enhance real-time processing capabilities. Quantum AI, while still in its infancy, promises to solve complex problems that are currently unsolvable with traditional computing.
However, these advancements come with challenges. Ethical AI, ensuring that AI systems are fair, transparent, and do not perpetuate biases, is becoming a critical area of focus. The privacy and security of data, as AI systems become more pervasive, will require innovative solutions. The job market will undergo significant changes, with some roles becoming obsolete while new ones emerge, necessitating a reevaluation of education and skill development.
Technical Breakdown: The Machine Learning Era
At the heart of many AI applications is machine learning, a subset of AI that enables systems to learn from data without being explicitly programmed. ML algorithms can be broadly categorized into supervised, unsupervised, and reinforcement learning, each suited to different types of problems.
- Supervised Learning involves training models on labeled data, where the algorithm learns to map inputs to outputs based on example inputs and their corresponding outputs.
- Unsupervised Learning deals with unlabeled data, where the model discovers patterns or relationships on its own.
- Reinforcement Learning involves an agent learning to behave in an environment by performing actions and seeing the results.
These approaches have been instrumental in achieving state-of-the-art results in image recognition, speech synthesis, and game playing, among other areas.
Decision Framework: Navigating AI Adoption
For individuals and organizations considering AI adoption, a structured decision framework can be beneficial:
- Identify Needs: Determine where AI can add value or solve pressing problems.
- Assess Feasibility: Evaluate the availability of relevant data and the technical capabilities required.
- Explore Options: Research different AI solutions and vendors.
- Pilot and Test: Start with a pilot project to gauge effectiveness and challenges.
- Deploy and Monitor: Gradually deploy the AI solution while continuously monitoring its performance and impact.
Conclusion
As Deirdre De La Cruz might ponder the complexities and wonders of AI, it’s clear that this technology is not just about machines; it’s about humans, our aspirations, and our limitations. AI represents a profound opportunity for growth, innovation, and improvement, but it also necessitates a thoughtful and responsible approach. By understanding AI’s evolution, current applications, future trends, and by developing frameworks for ethical and effective adoption, we can unlock its full potential to create a brighter, more equitable future for all.
What is the current state of artificial intelligence?
+Artificial intelligence is currently in a phase of rapid advancement, with applications in various industries such as healthcare, finance, and automotive. It is being used for automation, analytics, and to enhance human capabilities.
What are the future trends in AI?
+Future trends include the integration of AI with the Internet of Things (IoT), the development of edge AI for real-time processing, and the potential of quantum AI for solving complex problems.
How can one get started with adopting AI?
+Getting started with AI adoption involves identifying needs, assessing feasibility, exploring options, piloting and testing, and then deploying and monitoring AI solutions.